The Client
The client, a prominent traditional grocery retailer in the USA, boasts an impressive annual revenue of approximately $2 billion. With an extensive network spanning over 250 store locations across multiple states, they rank among the largest grocers nationwide. Their strategic positioning and expansive reach solidify their stronghold in the market, catering to diverse customer needs. Operating at such a scale underscores their commitment to delivering quality products and exceptional service, further cementing their reputation as a trusted destination for grocery shopping in the United States. They leveraged our grocery data scraping services to collect grocery price and performance insights, driving strategic decisions for sustained growth.
Key Challenges
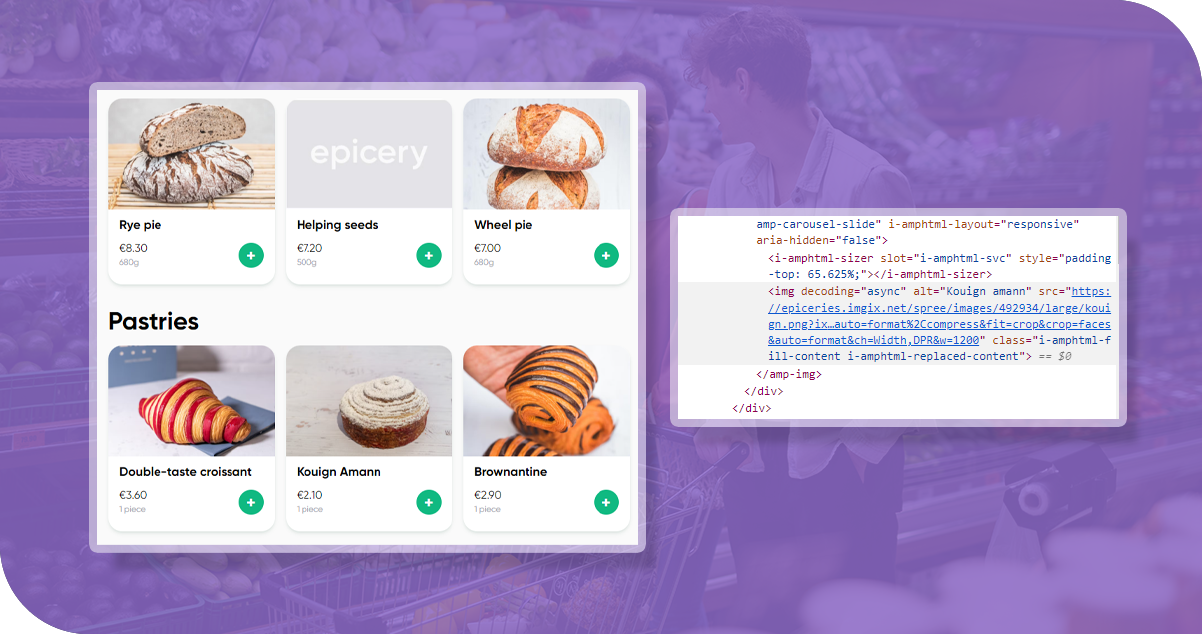
During our efforts to scrape grocery price data, we faced several notable challenges. Foremost among these was the task of accurately matching products against similar offerings from competitors, a process complicated by the diverse range of items and varying descriptors used across different sources. Additionally, tracking prices across multiple zip codes proved daunting due to discrepancies in regional pricing and availability. Another significant hurdle arose in comparing prices for fresh produce and non-branded SKUs, which often need standardized identifiers, making accurate matching and analysis more complex. Furthermore, the inability to effectively monitor competitor discounts and promotions hindered our client's ability to adjust their pricing strategies accordingly. Overcoming these challenges demanded meticulous attention to detail, innovative approaches, and advanced data analysis techniques to deliver precise, actionable insights to our clients.
Key Solutions
To address the challenges encountered while scraping grocery price data, we implemented the following solutions:
- Enhanced Matching Algorithms: We refined our matching algorithms to improve the accuracy of product comparisons across competitors. By incorporating advanced techniques, such as natural language processing and machine learning, we optimized the process of identifying similar products despite variations in descriptors.
- Geographic Mapping Tools: Utilizing geographic mapping tools, we streamlined the tracking of prices across multiple zip codes. It enabled us to account for regional pricing differences and provide more accurate insights into market trends and fluctuations.
- Customized Product Identification: We developed customized identification methods for fresh produce and non-branded SKUs to overcome the need for standardized identifiers. Through manual curation and categorization, we ensured precise matching and analysis of these items, enhancing the reliability of our data.
- Competitor Monitoring Software: We integrated specialized competitor monitoring software to track discounts and promotions run by competitors effectively. It allowed our client to stay updated on market trends and adjust their real-time pricing strategies, maintaining market competitiveness.
By implementing these solutions, we successfully overcame the challenges faced during the data scraping process, delivering accurate and actionable insights to our clients for informed decision-making and strategic planning.
Methodologies Used
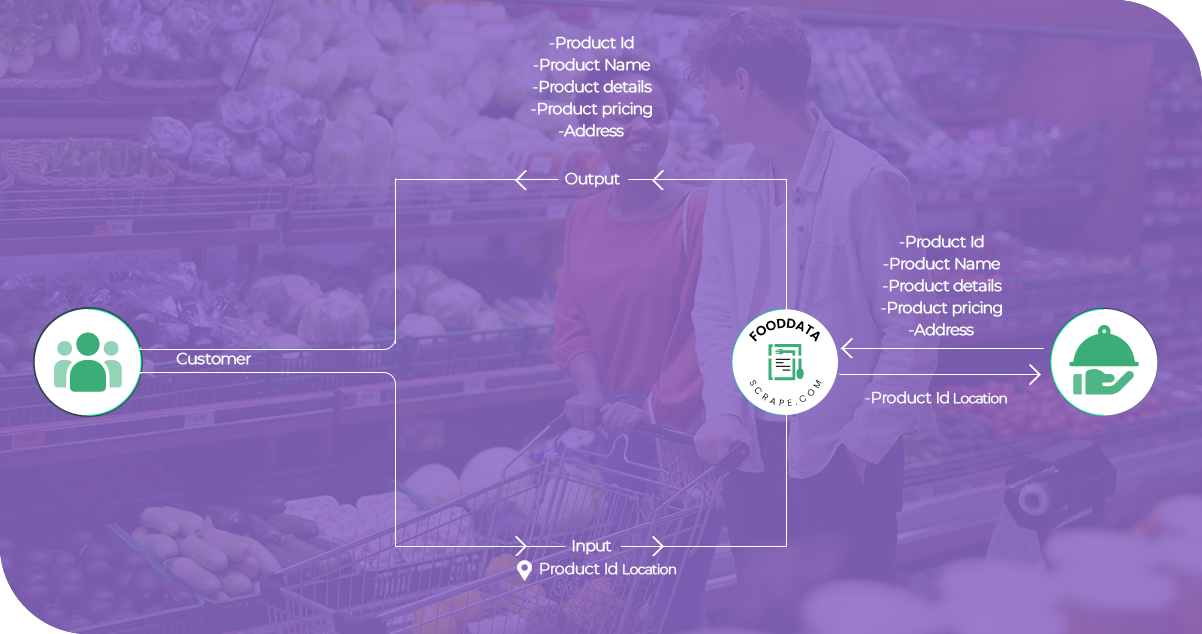
We employed a range of methodologies to scrape grocery product price data and conduct comparisons:
- Web Scraping: Employed automated web scraping tools to extract pricing information from diverse online sources, including retailer websites and e-commerce platforms.
- API Integration: Integrated application programming interfaces (APIs) provided by grocery retailers to access and retrieve real-time pricing data, ensuring accuracy and efficiency in comparisons.
- Data Aggregation: Gathered pricing data from various sources such as official retailer websites, online marketplaces, and third-party databases, aggregating them into a centralized dataset for comprehensive analysis.
- Machine Learning Matching: Utilized machine learning algorithms to match and align similar products across different retailers, facilitating automated price comparisons even for items with varied descriptions.
- Manual Verification: We conducted manual verification checks to ensure the accuracy and reliability of scraped data, cross-referencing prices, and product details across multiple sources to eliminate errors and discrepancies.
- Dynamic Monitoring Systems: Implemented dynamic monitoring systems to track price changes and promotional offers in real time, enabling proactive adjustments and timely insights into market trends and competitor strategies.
Advantages of Collecting Data Using Food Data Scrape
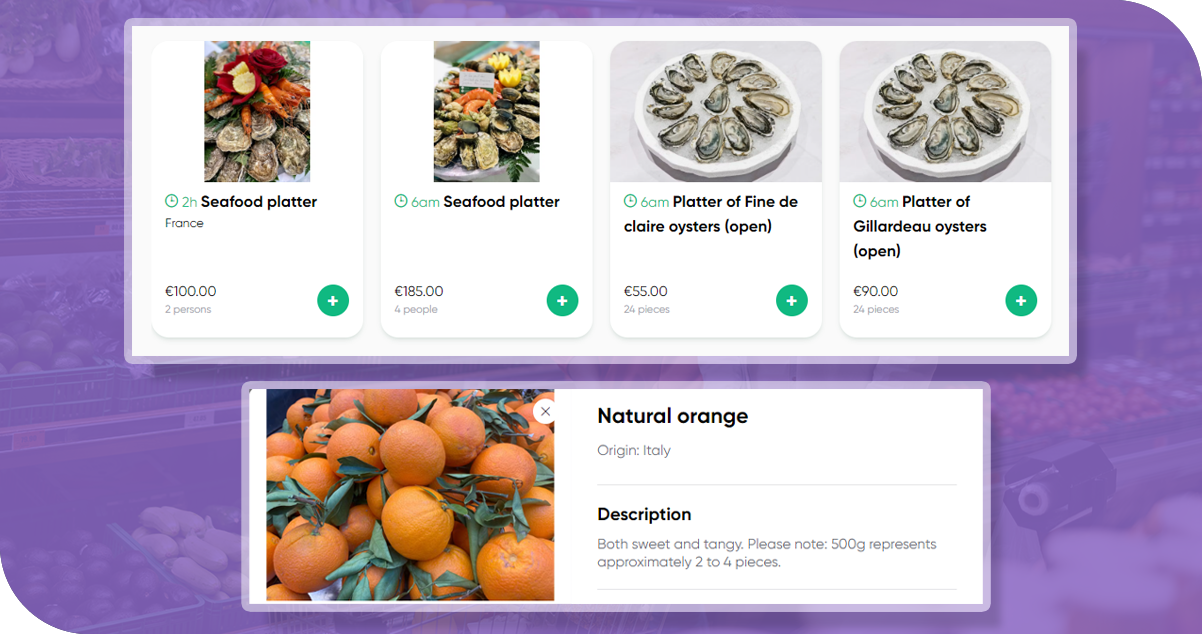
Comprehensive Coverage: With our high-velocity, high-volume scraping capabilities, we can efficiently handle large-scale data extraction, covering thousands of products across numerous competitors and zip codes, providing you with a comprehensive dataset for analysis.
Granular Insights: Our complete domain scraping approach allows for a detailed gap analysis of competitor catalogs, empowering you to make informed decisions on location-specific pricing and product assortments.
Precision Analytics: Leveraging zip code-level scraping and custom data templates, we deliver precise analytics tailored to your needs, enabling you to understand locality-based pricing differentials and make adjustments accordingly.
Partner Integration: By scraping third-party delivery partner websites like Instacart, we offer deeper insights into commissions and mark-ups, giving you a holistic view of your online delivery ecosystem.
Coupon Intelligence: Our grocery data scraper captures promotions, discounts, and coupons from various locations on websites, ensuring you have access to all available savings opportunities to optimize your pricing and promotional strategies.
Real-Time Updates: Our high-refresh-rate scraping provides up-to-date data regularly, empowering you to stay agile and respond quickly to market changes and competitor actions.
Tailored Solutions: We understand your unique scraping needs and offer customized solutions, whether high-velocity scraping, complete domain scraping, or zip code-level analytics, ensuring you get the insights you need to drive success in your business.
Final Outcomes: After scraping grocery price data, their pricing team improved competitive pricing, boosting sales volume by up to 4% from the second month onwards. They also enhanced pricing strategies for private label brands, resulting in a significant sales share increase of over 8% compared to national brands. It helped the procurement team leverage competitor pricing insights for better negotiations with national brands. Furthermore, the marketing team developed a robust promotion strategy aligned with competitors' tactics, utilizing scraped data to enhance promotional zoning at the zip code level.